上次国庆节去谷歌开发者大会,体验了很多有趣的人工智能项目。
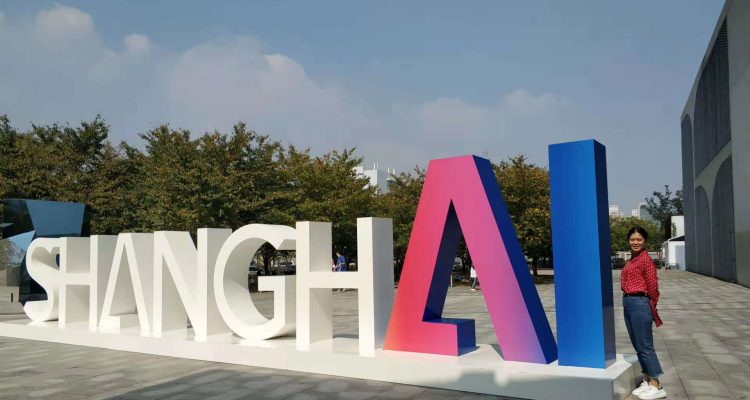
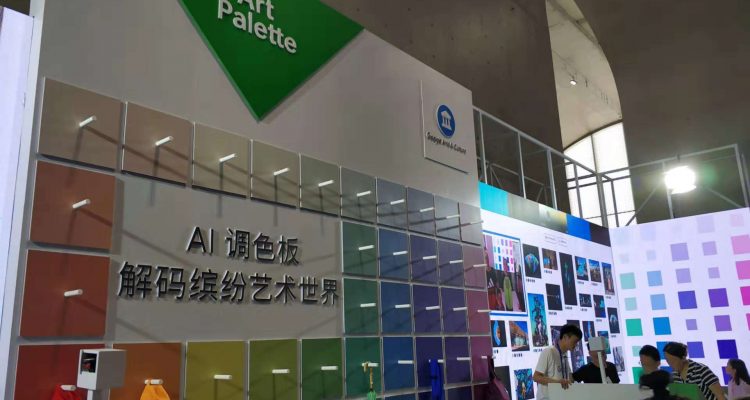
其中有一个颜色匹配的环节,叫做“AI调色板,解码缤纷艺术世界”,让我觉得很有意思,回来后,我计划自己实现一个类似的功能。
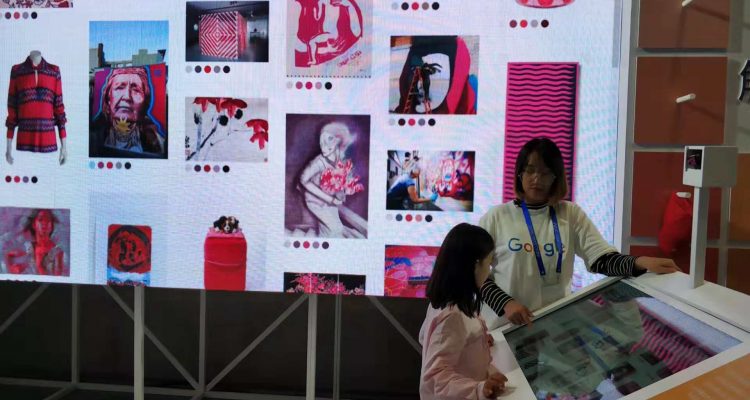
简单点讲,原理其实很简单,
- 给系统输入一张图片
- 系统检测输入图片中的什么色彩占比比较大
- 根据上一步识别的色彩,到庞大的图片库中匹配与之类似的图片
首先,我们需要想办法提取出图片中的主体颜色。这里我用了传统的机器学习算法——k-means来对图片上的像素值进行聚类。
from skimage import io
from sklearn.cluster import KMeans
import numpy as np
import warnings
warnings.filterwarnings('ignore')
img_file = 'city.jpg'
# k-means中的k值,即选择几个中心点
k = 5
# 读图片
img = io.imread(img_file)
# 转换数据维度
img_ori_shape = img.shape
img1 = img.reshape((img_ori_shape[0] * img_ori_shape[1], img_ori_shape[2]))
img_shape = img1.shape
# 获取图片色彩层数
n_channels = img_shape[1]
estimator = KMeans(n_clusters=k, max_iter=4000, init='k-means++', n_init=50) # 构造聚类器
estimator.fit(img1) # 聚类
centroids = estimator.cluster_centers_ # 获取聚类中心
# 使用算法跑出的中心点,生成一个矩阵,为数据可视化做准备
result = []
result_width = 200
result_height_per_center = 80
for center_index in range(k):
result.append(np.full((result_width * result_height_per_center, n_channels), centroids[center_index], dtype=int))
result = np.array(result)
result = result.reshape((result_height_per_center * k, result_width, n_channels))
# 保存图片
io.imsave(img_file + '.result.bmp', result)
程序里的city.jpg就是下面这张图片
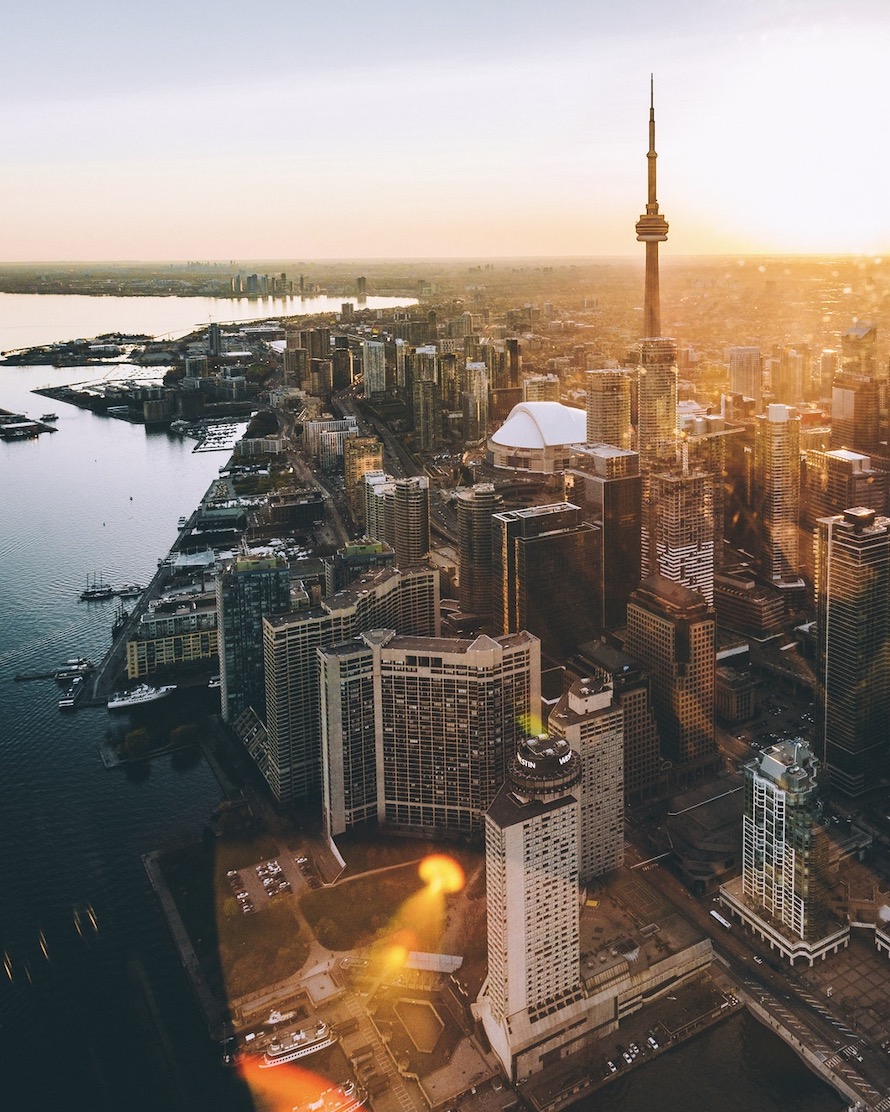
代码里,我们设置的k值是5,下面程序中计算出来的中心点,每个点由三个点构成,分别对应R,G,B通道。
- [41.1908632, 42.93972933, 42.17768105]
- [235.53604525, 229.25576283, 230.17416456]
- [196.38345078, 162.31462071, 129.28781508]
- [144.83752455, 113.68305275, 87.47028264]
- [89.70849154, 71.46176502, 58.98393717]
将这些数据进行可视化,得到下图。可以看出,分类的颜色基本上是city.jpg中占比比较大的颜色。
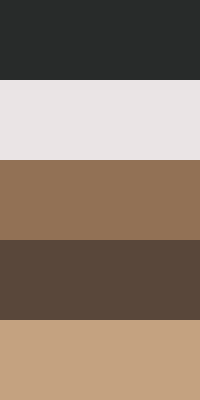
我们多运行几次试试看返回的颜色是不是会变化。
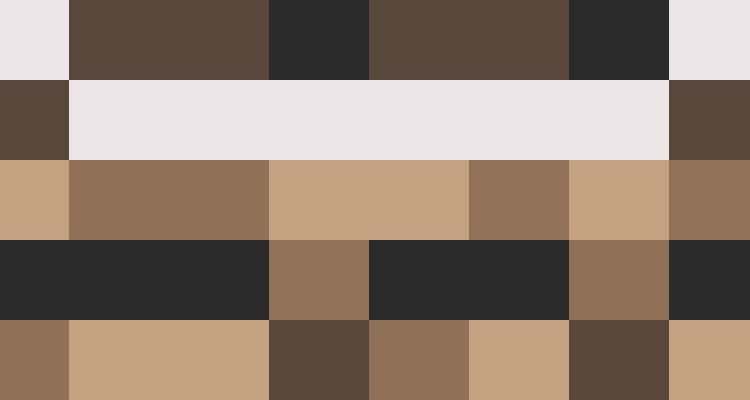
下次有时间把接下来的内容进行完善:
- 用python实现k-means,不依赖sklearn
- 将多张图片的主体颜色结果进行匹配,对相似颜色的图片进行聚类
此项目代码已经上传到github上了:https://github.com/shulisiyuan/mainColor
发表回复